Computer Science specialists from Princeton University published a study in which they showed that the use of AI often leads to errors that are extremely difficult to find. A lot of works in various fields of science use incorrect data to train networks. This leads to false predictions. Such errors are especially dangerous in medical applications.
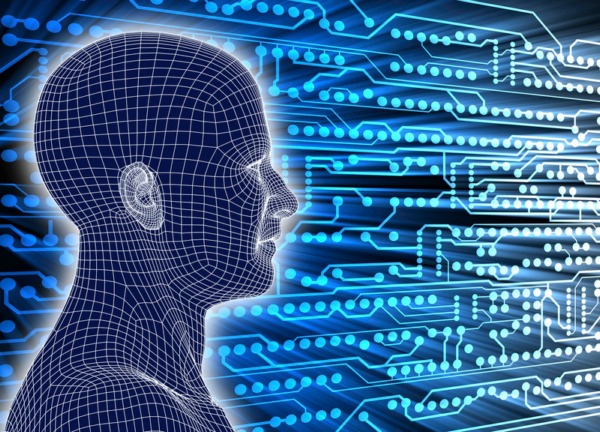
Today, AI methods and especially neural networks are used in a variety of fields of science — from genome research to the registration of rapid bursts in astronomy. Neural networks are very popular. They are somewhere at the very top of the hype among all scientific methods. A scientist who does not use them seems to be a retrograde, and one who actively uses them is a modern-advanced specialist.
But neural networks were developed to solve a rather limited range of tasks (primarily image recognition tasks). The founding fathers of this method were serious people, and they knew well how to use neural networks correctly.
And when neural networks began to be used by scientists very far from Computer Science in their specialization, there were irreproducible results that cannot be repeated, and incredible predictions that are simply false.
This was written in their study by scientists from Princeton University Sayash Kapoor and Arvind Narayanan. In his commentary on their work, Nature writes: a chemist scientist understands that it is impossible to seriously understand chemistry by listening to an online seminar, but for some reason he is sure that it is quite possible to understand the details and risks of using a neural network from such a seminar. This is at least strange. The neural network is not a push-button calculator after all.
What are we risking
The main risk when using neural networks is incorrect or incomplete preparation of data for training. As Kapoor and Narayanan write, not all scientists using neural networks can distinguish correct data for training from data that make a deliberate mistake.
Experts call one of the types of errors “data leakage”. The article provides the following example. In the work of economists, an attempt was made to predict stock exchange rates at the end of the day according to messages on Twitter. The result was simply stunning — the accuracy of the prediction exceeded 80%. Why did this happen? Kaput and Narayanan was explained. The course at the end of the day is not formed instantly. Of course, there may be jumps, but they are just about 20%. The remaining courses are formed more or less smoothly. And this smooth formation of courses is reflected in Twitter posts. That is, when the neural network “predicts” the result, it already “knows” it, because it received it from the training data. But you can’t train the network on the results you want to get. Then you will definitely get them, but there is no sense from it, besides, you will publish an article and everyone will be delighted.
Scientists also give examples of erroneous interpretation of the result. For example, the neural network perfectly recognizes a cow in the drawing. And let’s first make sure that it recognizes the cow, and not the meadow on which the cow is standing. So it may well happen if all the pictures on which we trained the network depict a cow standing in a meadow.
The main danger is mistakes in medical research. Scientists give such an example. If we trained a neural network to recognize lung diseases only on a group of elderly patients, then in no case can the same neural network be used without additional training to recognize the lungs of young people. This is simply dangerous, because the neural network will see what is not there and will give false recommendations.
Kapoor and Narayan have developed a protocol that allows you to verify the operation of a neural network. Scientists hope that specialists from fields far from computer science will adhere to this protocol, and at least partially will be insured against false predictions and errors.